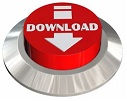

* Quote illustrates the point with it's absurdity. Good prediction is possible despite no structural relationship

Leinweber was able to "predict" past U.S. cheese production and the total population of sheep in both Bangladesh and the U.S., Mr. Over an 13-year period, found, "explained" 75% of the variation in the annual returns of the Standard & Poor’s 500-stock index.By tossing in U.S. * Random Forests Boosting Deep Learning - non-parametric and hard to interpret as explanatory. * Ridge regression - no more confidence intervals p-values * Statistical Models often predict well, even if they are not built up from a realistic model of the world.
Sims 4 model career 1.25 how to#
* Familiar to most in ML: how to understand evaluate predictive models. * I've been teaching undergraduates from these familiar texts.

* Not the first, nor will we be the last to point out the flaws, but the models are still terrible. * I'll describe the details more later, but suffice it to say that this work started 10+ years ago, and still isn't even a completed working paper. * The idea that a certain mathematical explanation for a real-world phenomenon is somehow "satisfying" when it incorporates as many of the underlying factors as possible. * Connects to the themes of this workshop well, I think. # "Beauty" or perhaps "elegance" as a research goal * Cosma originally scheduled, but couldn't make it. * Thanks to the organizers for the invitation # NeurIPS: I Can't Believe It's Not Better Your model is beautiful, but does it predict?
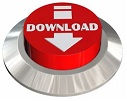